Federated Recommender Systems for News Articles
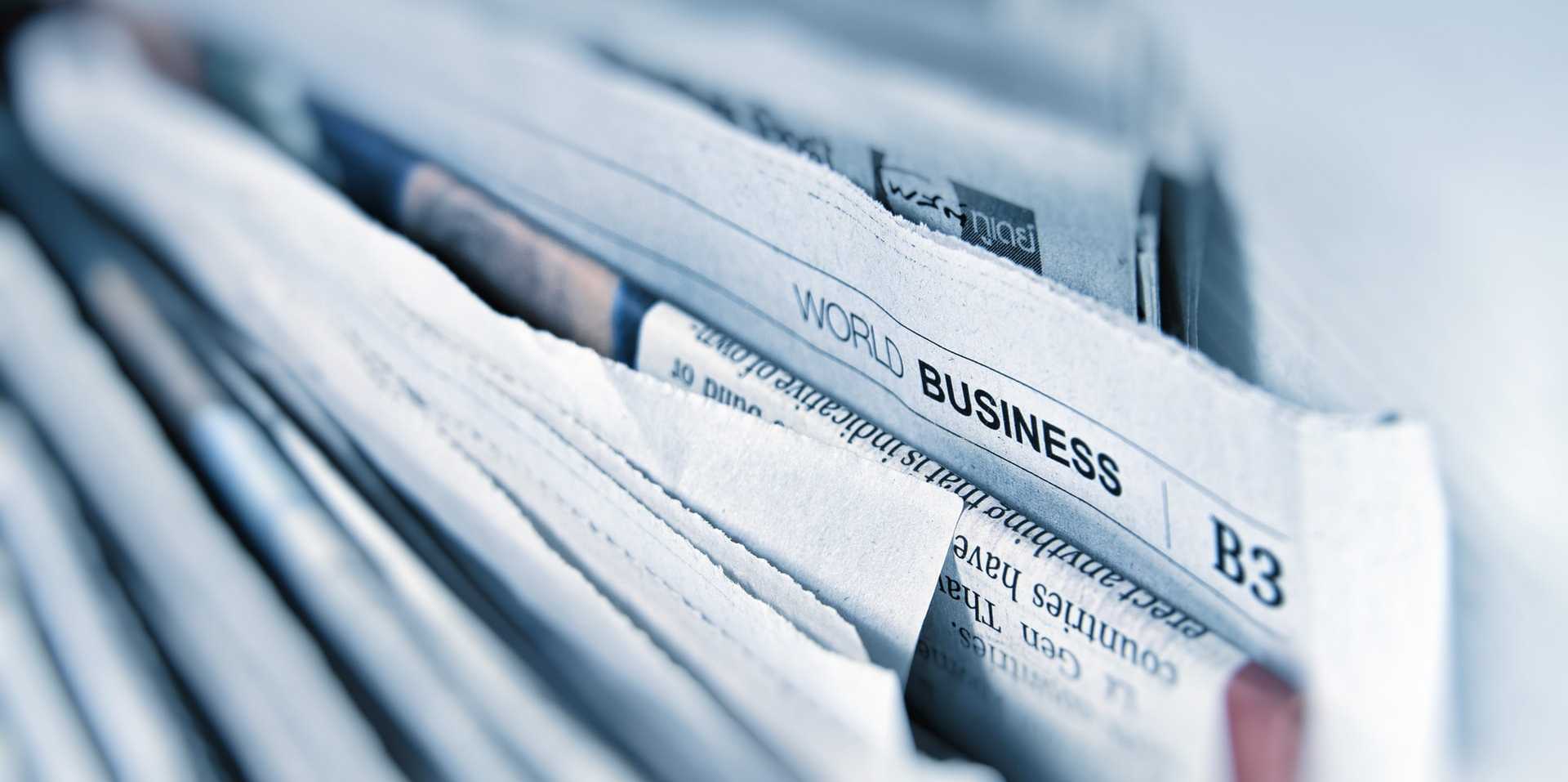
Project description
Recommender systems completely rely on the knowledge of training information to generate recommendation for users. The training information in the form of user ratings or clicks are widely used to model a user's interest and generate recommendations. However, the training information is not easily accessible or shared to a recommender in order to ensure privacy, ownership and protection to user information.
Federated learning is widely explored to learn a shared model from millions of mobile devices (each mobile device corresponds to single user data). However, for RSs, there are many scenarios, where each client can be, for examples, hospitals, banks, restaurants who hold data from many users but cannot exchange their information to boost their training information. In this project, we consider such scenarios where the clients are a small number of organizations or industries collaborating to learn shared recommendation models.
Marco Schöb
Bachelor's Thesis
Status:
Finished
Acknowledgments:
The author of the following paper would like to acknowledge that Mr. Marco Schöb contributed to the experiments by applying a filter on the test set using our codes, our experiment setup, and our datasets. We thank Marco for the support.
Kalloori, Saikishore. “Federated Learning for Cold Start Recommendations.” Proceedings of the 7th Joint International Conference on Data Science & Management of Data (11th ACM IKDD CODS and 29th COMAD). 2024