Towards Federated Learning Algorithms for Online Media Systems
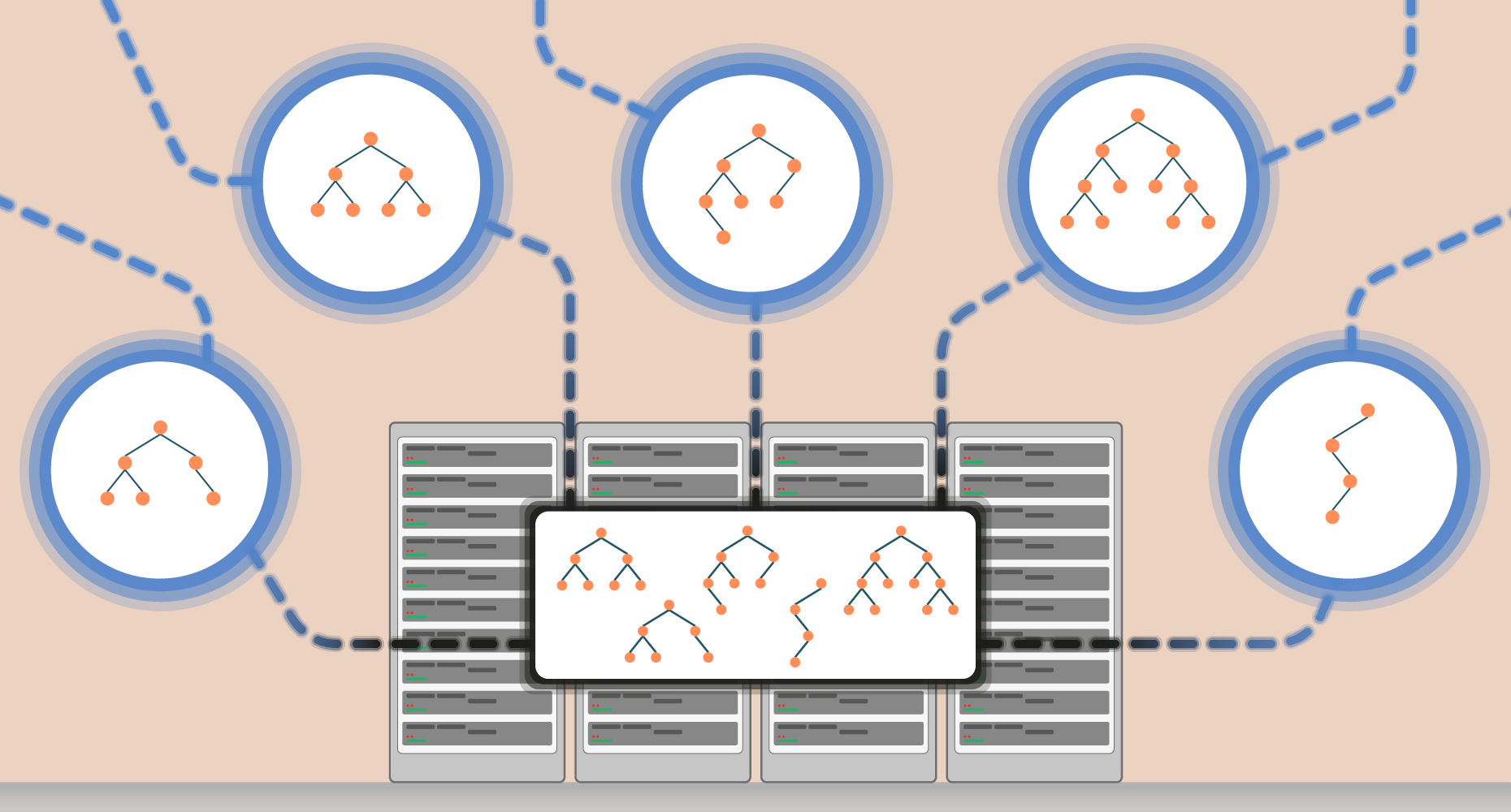
Abstract
Federated Learning is a machine learning technique that allows a machine learning model to be trained on data from several servers with useful information without exchanging the data to another server. Such an approach is becoming increasingly important in a world with exponentially growing data and a desire for data protection. In this thesis, we propose three algorithms that allow training a random forest in a federated learning setup. We then perform an experimental analysis of these algorithms based on four data sets to determine their properties with respect to improving the performance of a random forest trained using local data. The results suggest that we can achieve an improvement in some metrics by applying our method compared to random forest models trained on local data only.
Roy Schubiger
Bachelor's Thesis
Status:
Completed