Anti-recommendation Engine for News Articles
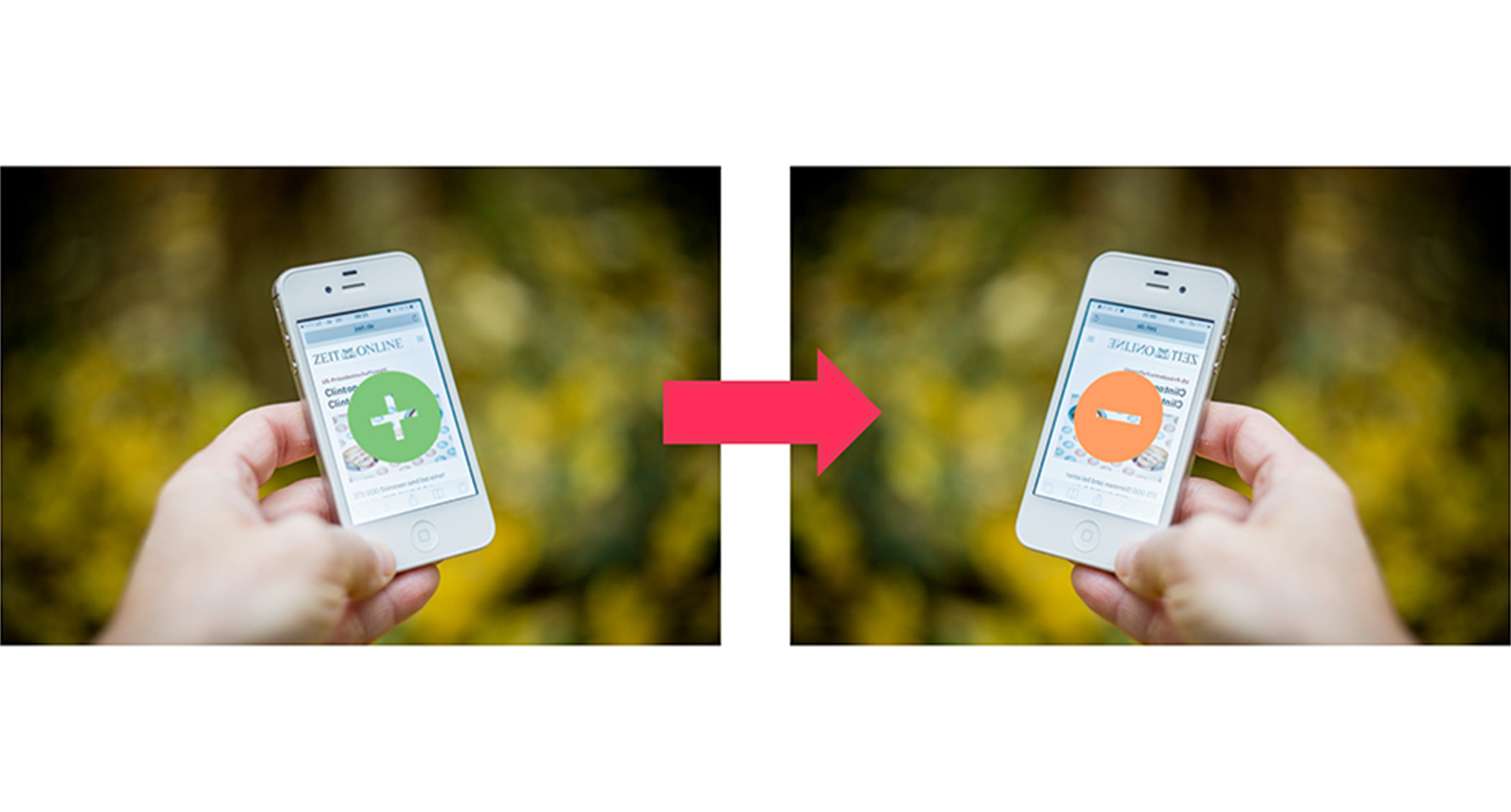
Abstract
Digitalization has lead to very personalized content and with it filter bubbles are formed. Escaping the bubble is hard because filter bubbles make it more difficult to access information outside of already established knowledge and interests. To counter this we try to find arguments with a different stance towards a topic and offer the option to an informed view in that matter.
We present the ProCon Dataset, a new argument pair dataset helping to combat the filter bubble. The dataset is composed of 35’000+ samples consisting of one question, two arguments and a label denoting if both arguments argue for the same side of the question or not. It is based on arguments from ProCon.org . Instead of fighting the filter bubble by proposing articles or arguments with opposing views we focus on the underlying task detecting if two arguments talk about the same topic but from a different side. Unlike other systems like IBM Debate we try solving this task only with pairs of articles instead of using a precomputed data structure storing information about topics. By only focusing on text features transferring a trained model to new topics is more likely to work because knowledge about the topic is not necessary. We describe multiple candidate models for solving this task leading to a baseline model with a validation accuracy of 65%. Human performance although is around 25% higher making the ProCon Dataset a challenging task for future research. Furthermore we are able to reach new state of the art results comparable to human performance on the FNC-1 Dataset .
Lucas Brunner
Bachelor's Thesis
Status:
Completed