Emotion and Stance Detection for German Text
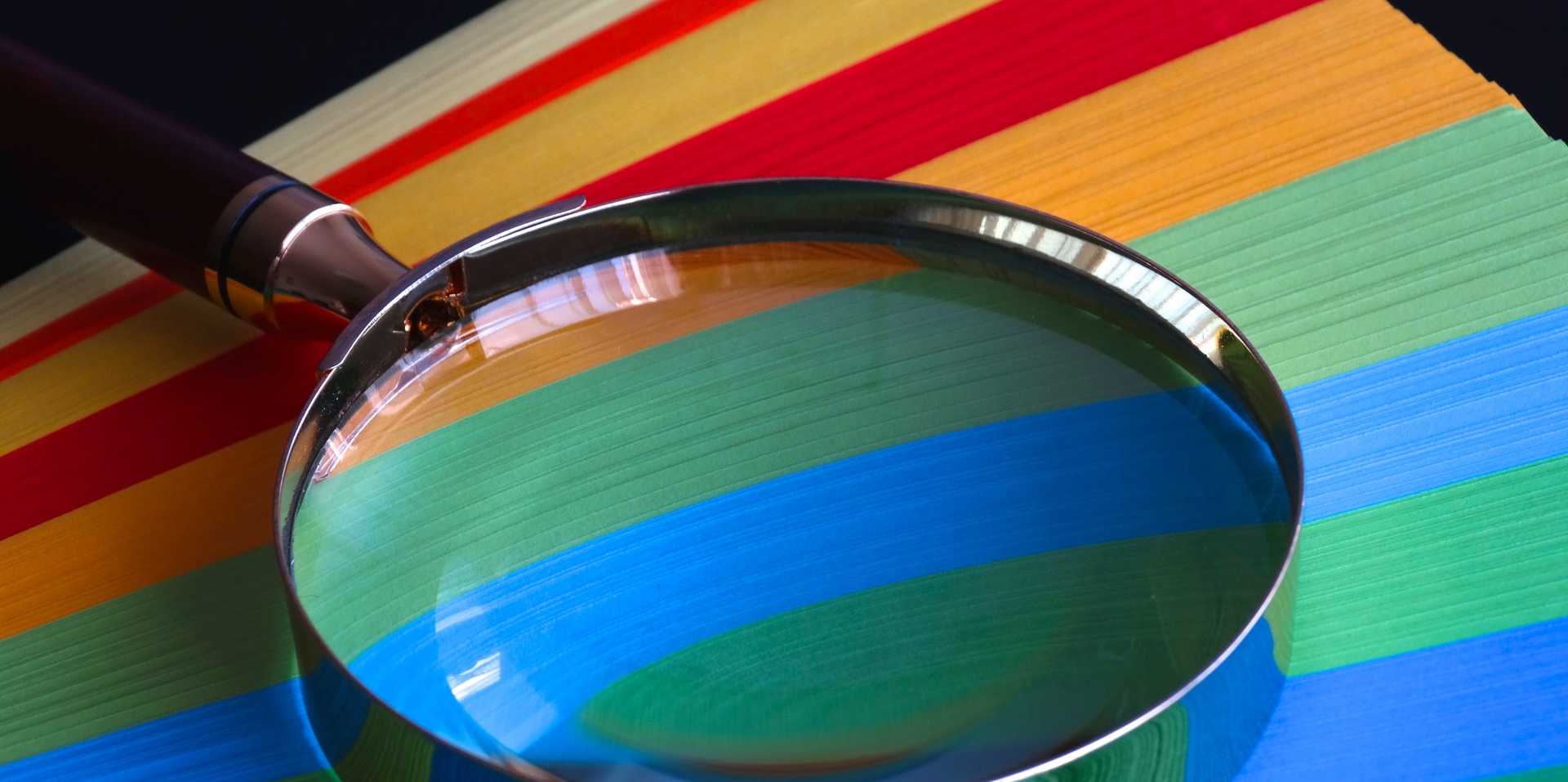
Emotion and stance detection can help to better curate produced content from journalists and users and can allow for a better understanding of reactions, behaviours, opinions and feelings of readers towards produced content. Furthermore, information about the stance or emotions conveyed in a text can provide additional insights into user engagement metrics, help with estimating propensities of users to register or subscribe, or potentially even open up new opportunities in advertising. While technologies for estimating the emotion and stance in text for English have seen large advances in the past years, these technologies lack behind for texts in German. In this project, we want to perform basic research on stance and emotion detection for German opinion texts. The goal is to close the performance gap between methods for English and methods for German.
Goals
Generate a high-quality data set. There is a lack of labeled datasets for German texts. This is why the first part of this project will focus on generating a high-quality dataset of journalistic text and comments to detect stance and emotions. For this we will use an appropriate crowdsourcing setup informed by previous work on crowdsourcing similar tasks. We will work closely together with journalists and editors to ensure that the labels provided by the workers are accurately reflecting how media professionals would rate such texts.
Shared Task for text classification. Based on the data collected, we will build baseline models for the different tasks using state-of-the-art methods. The data together with the baseline models will be released as a shared task to the research community. We expect that a data set for stance detection will be especially interesting to the community. We will provide a special test data set that challenges methods to generalize across debates and topics.
Outcome
1. As the first step, we have built an annotation tool that can enable the annotators to provide a stance label for a given question and articles and a emotion label for a given article text on paragraph level and full-article level. Our annotation tool has simple UI and functionality to elicit the emotions and stance labels.
2. Using our annotation tool and with post processing methods, we created the CHeeSE dataset: The German Dataset of Swiss (CH) News Articles for Stance and Emotion Detection consists of:
- Data
- 91 debate questions
- 670k Swiss news articles (Blick, NZZ, NZZaS)
- 3’693 annotated question-article pairs
- Annotations
- Stance, Paragraph Emotion, Article Emotion
3. We further released the dataset for the research community and the detail can be found in the below publication ‘Stance Detection in German News Articles’
4. We built machine learning models and performed offline experiments on the CHeeSe dataset for both detection tasks.
Follow Up Project Extension
Goals
In the follow up extension, we aim to develop end-user prototypes that demonstrate the functionality and possibilities of our emotion and stance detection models. For this, we build an emotion viewer tool, which, for a given text from a news article, will predict the emotions that the text conveys. We build a debate portfolio prototype that displays articles grouped by their stance. For example, given the topic “exit nuclear energy”, the user will obtain an overview of news articles that are "in favour", "against", or "discussing" the topic. We are also exploring the usage of emotions for recommender systems where we aim to incorporate the user and articles expressed emotions into a recommender system model and generate accurate news article recommendations.
Outcome
1. We built an emotion viewer tool and stance viewer tool using the dataset we created. The emotion viewer, for a given text from a news article, will predict the emotions that the text conveys. The stance viewer displays articles grouped by their stance. For example, given the topic “exit nuclear energy”, the user will obtain an overview of news articles that are "in favour", "against", or "discussing" the topic.
2. We designed models to exploit the usage of expressed emotions from the article text for the recommendations of news article.
- We conducted experiments using NZZ data with 38K users, 6K news articles and 7million user-article impressions. Our recommendation models includes Content based CF model, Factorisation machines (FM) and Deep Neutral FM Model (DeepFM).
3. Our experiments and analysis demonstrate that usage of expressed emotions from articles boost the recommender systems performance.
- Specifically, usage of raw (extracted) features is not beneficial. For instance, when raw emotions are added into a content-based model, we noticed decrease in the ranking performance.
- Modelling text and emotions in a linear combination does not yield good performance.
- Higher order modelling model like factorisation machines and deep factorisation models demonstrated good benefits in the ranking performance.
4. We conducted experiments to understand when does using expressed emotions show a major benefit. Our results suggest that for users (semi-cold start users) with few ratings available to the recommender system, exploiting the emotions have shown significant improvement in ranking when compared to a model that do not exploit emotions during recommendation. We also conducted a qualitative analysis, and our results demonstrate when using our emotion aware recommender system, we found that from the top 4 recommendations, more than 98% match at least one emotion from the user’s previous read articles.
Publications
Public Open-Source Repositories
CHeeSE Dataset
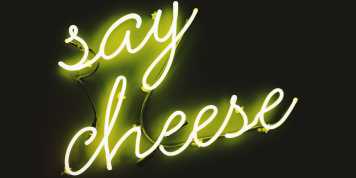
A collection of manually annotated Swiss news articles in German, where each pair of news articles and debate questions is annotated with the stance of the article towards the question, the article emotion, and the emotion of each individual paragraph. Find out more
Online Text Labelling
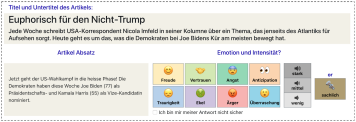
As part of our emotion & stance project we developed a streamlined web app to collect text labels. The tool was developed for news article annotation but can be easily adapted for different use cases. Find out more
Additional Project Resources
Resources for Industry Partners
Additional project resources for our industry partners are only available to registered users and can be found here.