Edit Propagation for Videos
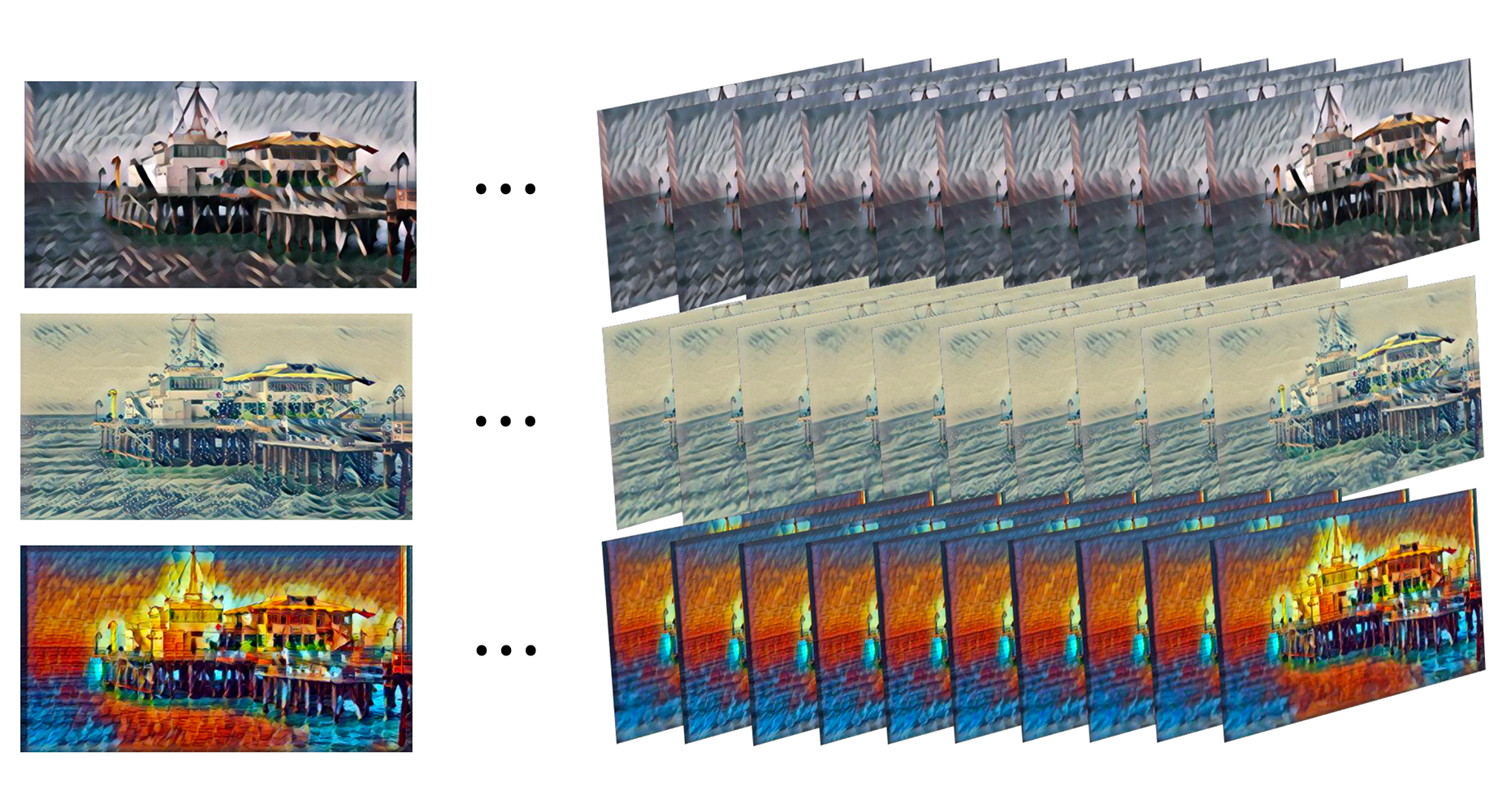
Abstract
Editing the visual style of a video manually can be a tedious task to ensure spatial quality and temporal stability. Most current approaches for artistic stylization of video are derived from deep-learning-based techniques for artistic style transfer for images. Their results can lack temporal consistency and are often difficult to influence resulting in a lack of artistic control. This means that desired changes might still have to be applied manually by artists on the whole resulting video sequence.
In this thesis we propose a deep learning based pipeline that propagates the style of an arbitrarily stylistically edited keyframe of a video sequence onto its subsequent frames. As input only the original video sequence and the edited first frame is required. In our pipeline we create multiple warps of the edited keyframe, some preserving local features, others global features, then combine and refine them. This allows the initial keyframe to either be edited using any of the many automatic artistic style transfer techniques, or directly by artists, allowing for significantly more artistic control over the end result.
Jan Wiegner
Bachelor's Thesis
Status:
Completed