Aesthetic Assessment of Image and Video Content
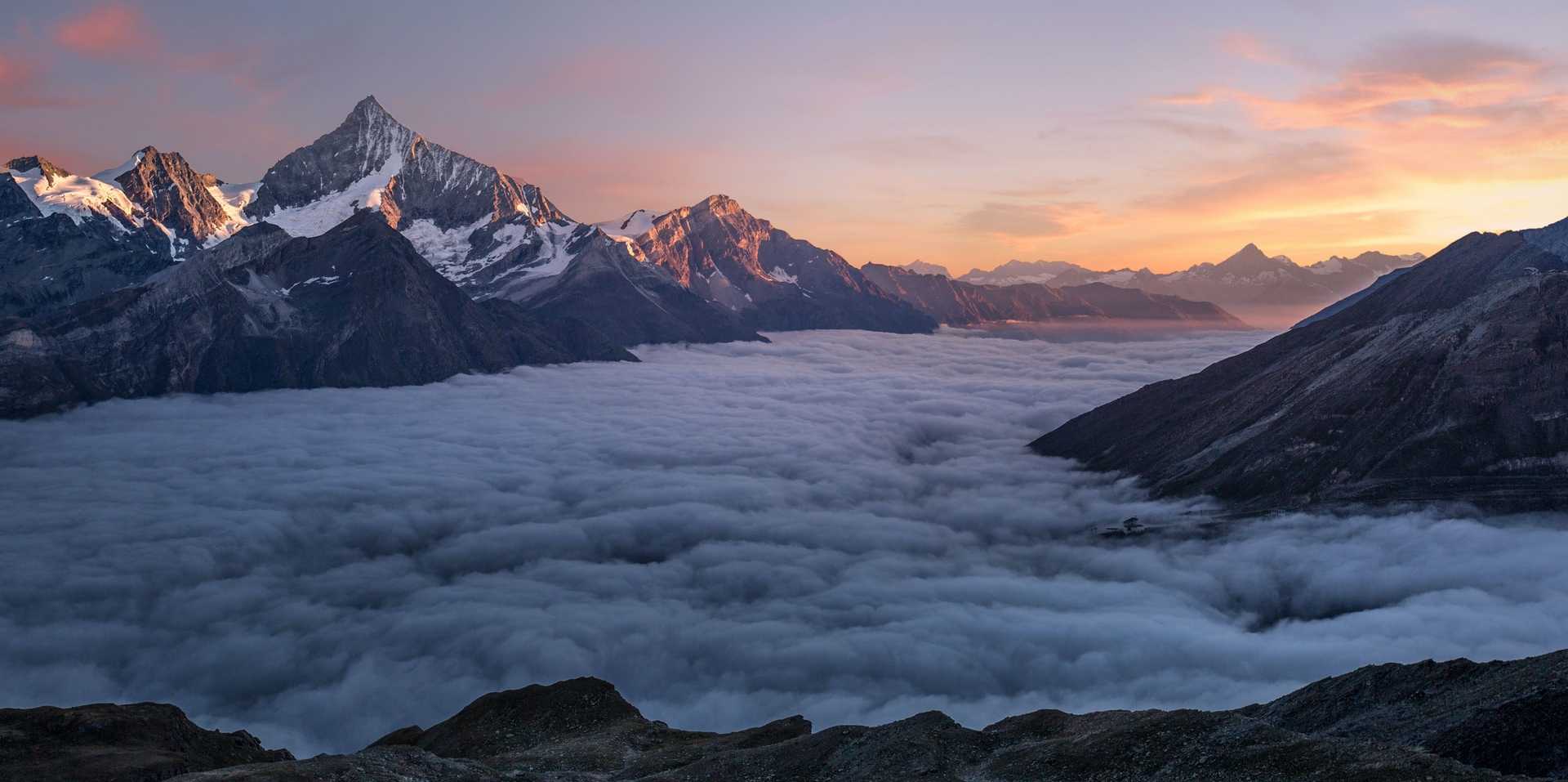
Images and videos are the ‘world’s visual language’ and have become the visual backbone of advertising and journalism. Therefore, the imaging style of the newspapers, magazines and TV shows is the result of very precise and finely tuned aesthetic choices. For this reason, we are interested in answering the challenging subjective question of "what makes images and video shots aesthetically pleasing?", which not only involves photographic and cinematic principles, but also how images are produced and what they say. Right now, selecting the best shots from thousands of hours of video material or selecting the perfect teaser image from a huge collection of images, according to these aesthetic principles, is a difficult and very time consuming task. In this project we devise methods for the automatic computation of image and video aesthetics given a user's intent.
Goals
The main goals of this project are threefold:
Build a unified image annotation tool to automatically label images and video shots with photographic, cinematic, aesthetic, semantic and technical features. The most relevant features were selected together with our industry partners. While many of the features can be extracted using existing state of the art methods, we found some exceptions and we observed that these methods use vastly different model structures which makes it hard to combine and expose such parameters to users
Integrate the annotation system into two use cases, to explore the robustness of the approach and the feasibility/performance of the system.
- Intelligent image search, sorting images by their aesthetic score.
- Automatic video summarization or keyframe/ shot selection.
Build an intelligent system to predict editor selected shots/images based on the features from step 1. We will use data from editor’s picks to train and evaluate our system. By analyzing a large dataset of video preview/thumbnails and promo material as well as selected images, we will be able to calibrate the scoring function for certain scenarios (e.g. action trailer, documentary preview etc.).
Outcomes
During this first period, we tested the best existing models to predict most of the proposed features and implemented some of the missing models. Additionally, we built a prototype of the framework that will support the unification of all the features.
In parallel, and in order to build a predictive model that is capable of imitating the decisions of the editors, we are building a labeling tool to obtain samples of how editors perceive image aesthetics. The results will be used to train a deep learning model that is already in construction and will help to sort images by their aesthetic score.
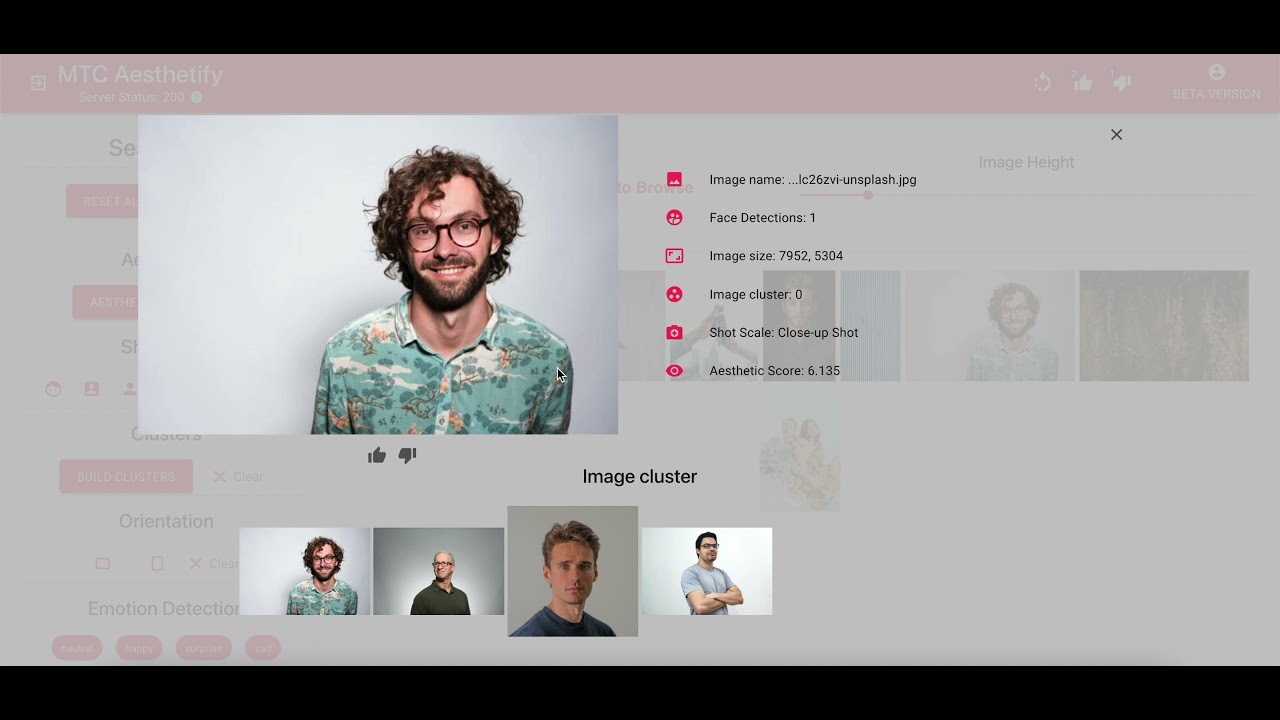

Public Open-Source Repositories
Tournament-style image rating tool
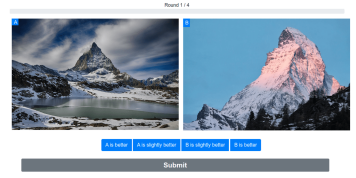
We developed a simple-to-use web tool to collect image rankings from users powered by a Swiss tournament style system. Relative user preference (one image over the other) are used to build a global ranking. Find out more
Interactive Post-editing Framework
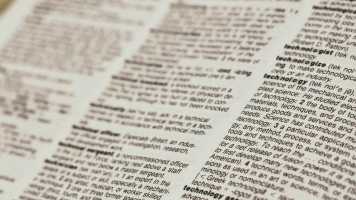
An open-source translation framework for interactive post-editing research. external page Find the repository on GitHub
Reddit Photo Critique Dataset (RPCD)
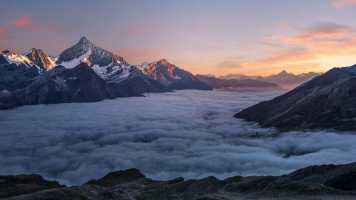
A dataset for aesthetic assessment that contains tuples of image and photo critiques. Find out more
Additional Project Resources
Resources for Industry Partners
Additional project resources for our industry partners are only available to registered users and can be found here.
Project Demo Applications
Project demos are hosted on our project demo external page dashboard. They are accessible by registered users only.