Long-term Visual Localization
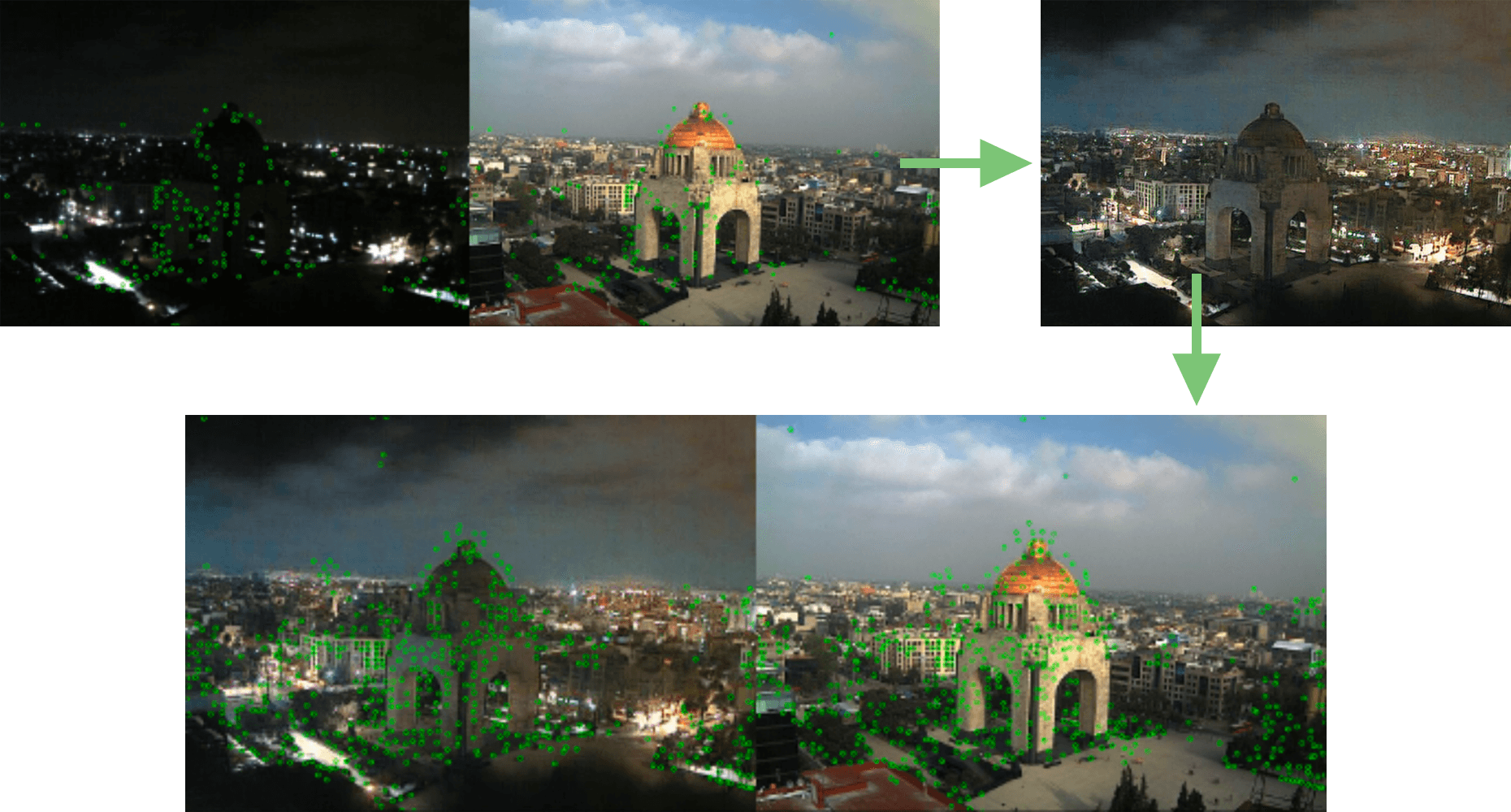
Abstract
This thesis addresses the problem of long-term visual localization, where the camera pose of a query image has to be found within a reference reconstruction of a scene. The biggest challenge of this task is to handle the various changes in illumination, weather conditions, seasons or viewpoints between the query and reference images. Convolution Neural Networks are the state-of-the-art for computing robust local features, but they are still insufficient for achieving precise localization of images exhibiting adverse conditions. Generative models have been developed to reduce the illumination gap by translating query night images into day. However, these models often rely on expensive training data, such as perfectly aligned images of a scene captured under different illumination conditions. Moreover, these generative models also need to train on query images, making them impractical for most real-world scenarios.
To remedy these issues, we propose an image transformation network that improves the performance of CNN local features when matching day and night images. In particular, we translate night images into day in a way that preserves or improves the resulting local features. Our approach can handle any illumination condition and does not need any training data, which is a novel contribution. We evaluate the model on image matching and visual localization tasks and show promising results in both cases.
Ali Uzpak
Master's Thesis
Status:
Completed