Adaptive Augmented Reality Assistant
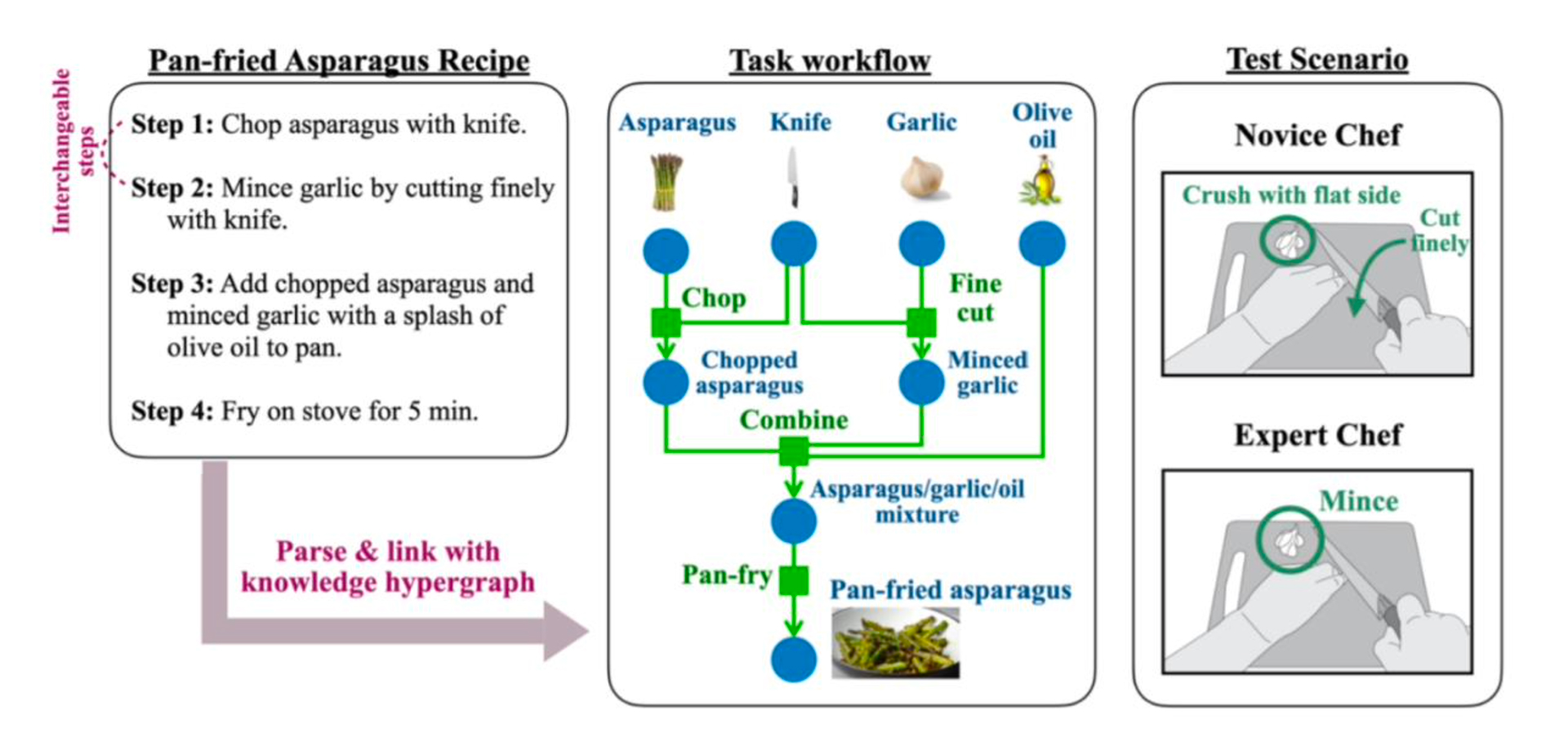
Project description
Self-guided tutorials are popular resources for learning new tasks, but they lack important aspects of in-person guidance like feedback or personalized explanations. Adaptive guidance systems aim to overcome this challenge by reacting to users’ performance and expertise, and adapt instructions accordingly. We aim to understand what users’ preferred balance of automation and control is, what representation of instructions they prefer, and how human experts give instructions to match users’ needs. We contribute two experiments where users perform different virtual and physical tasks, guided by instructions that are controlled by experts using a wizard-of-oz paradigm. We employ different levels of automation to control instructions and alter the representation of the Augmented Reality instructions for the physical task. Results indicate that while users preferred automated systems for convenience and instant feedback, they appreciated a degree of manual control since they felt less rushed. Experts relied on factors such as expected expertise, hesitation, errors, and their understanding of the current task state as main triggers to adapt instructions. Furthermore, users preferred AR instructions that were offset from the physical task, to balance seeing instructions close and in great detail while avoiding visual interference. We believe that our findings and leveraging expert behavior as a principled approach inform the design of future adaptive guidance systems.
Robin Wiethüchter
Master's Thesis
Status:
Completed